Edge AI for Delivery Optimization
Edge AI for Delivery Optimization uses real-time data processing at the edge to enhance efficiency and speed of delivery operations.
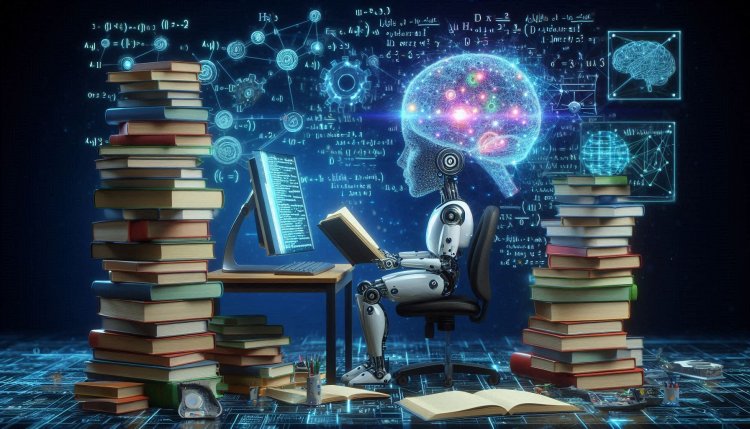
Edge AI for Delivery Optimization
In recent years, the rise of e-commerce and the increasing demand for fast and efficient delivery services have put pressure on companies to optimize their delivery processes. One of the key technologies that have emerged to address this challenge is Edge AI, which combines artificial intelligence with edge computing to enable real-time decision-making at the edge of the network. This technology has the potential to revolutionize the way companies manage their delivery operations and improve efficiency, speed, and customer satisfaction.
What is Edge AI?
Edge AI refers to the deployment of artificial intelligence algorithms and models on edge devices, such as sensors, cameras, and other IoT devices, rather than on centralized servers or in the cloud. This allows data to be processed and analyzed locally, near the source of generation, enabling real-time decision-making without the need to send data back and forth to a centralized server. Edge AI is particularly useful in scenarios where low latency and real-time processing are critical, such as in delivery optimization.
Benefits of Edge AI for Delivery Optimization
There are several key benefits of using Edge AI for delivery optimization:
- Real-time Decision Making: Edge AI enables real-time analysis of data at the edge of the network, allowing companies to make quick decisions based on the most up-to-date information. This is crucial for optimizing delivery routes, managing inventory levels, and responding to changing customer demands.
- Reduced Latency: By processing data locally on edge devices, Edge AI reduces the latency associated with sending data to a centralized server for processing. This results in faster response times and improved delivery speed, which is essential for meeting customer expectations for fast and on-time deliveries.
- Improved Efficiency: Edge AI can help companies optimize their delivery routes, predict demand patterns, and automate various aspects of the delivery process. This leads to increased efficiency, cost savings, and reduced carbon footprint by minimizing unnecessary trips and idle time.
- Enhanced Security and Privacy: Edge AI enhances security and privacy by processing sensitive data locally and minimizing the need to transmit data over the network. This reduces the risk of data breaches and ensures compliance with data protection regulations.
Applications of Edge AI in Delivery Optimization
Edge AI can be applied in various ways to optimize delivery operations:
- Route Optimization: Edge AI algorithms can analyze real-time traffic data, weather conditions, and delivery constraints to optimize delivery routes for speed and efficiency. This can help companies reduce delivery times, fuel consumption, and operational costs.
- Dynamic Inventory Management: Edge AI can monitor inventory levels in real-time and predict demand patterns to optimize inventory replenishment and prevent stockouts. This ensures that products are available when needed and minimizes excess inventory holding costs.
- Customer Demand Prediction: By analyzing historical data and customer behavior in real-time, Edge AI can predict future demand patterns and adjust delivery schedules and inventory levels accordingly. This helps companies meet customer expectations and reduce the risk of overstocking or understocking.
- Automated Delivery Vehicles: Edge AI can be used to automate delivery vehicles and drones, enabling autonomous navigation, obstacle avoidance, and route planning. This reduces the need for human intervention and improves delivery speed and accuracy.
Challenges and Considerations
While Edge AI offers significant benefits for delivery optimization, there are also challenges and considerations that companies need to address:
- Scalability: Companies need to ensure that their edge devices can scale to support the increasing volume of data generated by delivery operations. This requires robust infrastructure and connectivity to handle the growing demand for real-time processing.
- Data Quality and Integration: To derive meaningful insights from data, companies must ensure the quality and accuracy of the data collected from various sources. Integrating data from different systems and devices can be complex and requires standardized formats and protocols.
- Algorithm Performance: Developing and deploying AI algorithms on edge devices requires optimization for performance, memory, and power consumption. Companies need to choose algorithms that are efficient and can run effectively on resource-constrained edge devices.
What's Your Reaction?
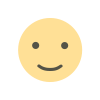
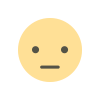
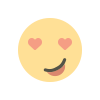
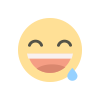
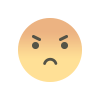
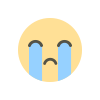
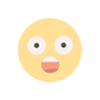