AI Bias Mitigation Techniques
Learn how to address AI bias with techniques like data preprocessing, algorithm transparency, diversity in training data, and continual monitoring.
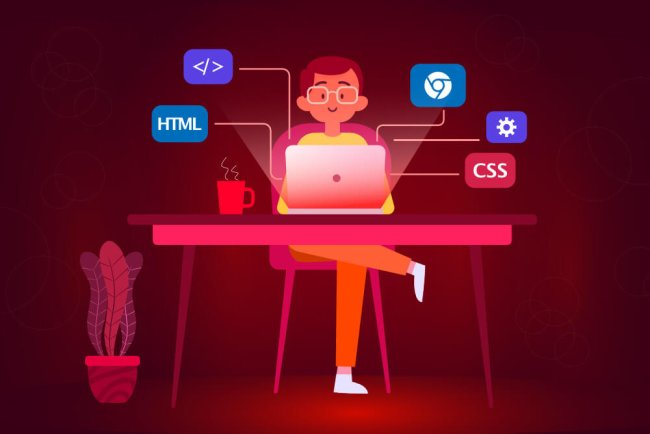
AI Bias Mitigation Techniques
Artificial Intelligence (AI) systems are increasingly being used in various sectors, but these systems can be prone to biases that can lead to unfair outcomes. It is crucial to employ techniques to mitigate bias in AI to ensure fairness and equity. Here are some key AI bias mitigation techniques:
1. Data Collection and Preprocessing
One of the main sources of bias in AI systems is biased data. It is essential to carefully collect and preprocess data to ensure that it is representative and free from biases. Data should be diverse and inclusive, representing different demographics and perspectives. Data preprocessing techniques such as data augmentation and oversampling can help mitigate bias in the training data.
2. Fairness-aware Algorithms
Developing fairness-aware algorithms is crucial for mitigating bias in AI systems. These algorithms are designed to explicitly account for fairness considerations during the training process. Techniques such as fairness constraints, bias-aware regularization, and adversarial learning can be used to promote fairness and reduce bias in AI models.
3. Bias Detection and Monitoring
Regularly detecting and monitoring bias in AI systems is essential for addressing bias issues. Bias detection techniques such as statistical parity, disparate impact analysis, and fairness metrics can help identify bias in AI models. By continuously monitoring for bias, organizations can take proactive measures to mitigate bias and ensure fairness.
4. Explainability and Transparency
Ensuring the explainability and transparency of AI systems can help mitigate bias by allowing stakeholders to understand how decisions are made. Techniques such as model interpretability, feature importance analysis, and explanation generation can provide insights into the factors influencing AI decisions. Transparent AI systems can help identify and address bias more effectively.
5. Diversity and Inclusion in Development Teams
Promoting diversity and inclusion within AI development teams can help mitigate bias by bringing diverse perspectives and experiences to the design and development process. Including individuals from different backgrounds can help identify and address biases that may not be apparent to a homogenous team. Diverse teams can contribute to building more fair and equitable AI systems.
6. Bias Impact Assessment
Conducting bias impact assessments can help organizations understand the potential impacts of bias in AI systems. By evaluating the potential harm or discrimination that biased AI systems may cause, organizations can take proactive steps to mitigate bias and ensure fairness. Bias impact assessments can inform decision-making processes and help prioritize bias mitigation efforts.
7. Human Oversight and Intervention
Integrating human oversight and intervention into AI systems can help mitigate bias by allowing human experts to review and intervene in AI decisions. Human-in-the-loop systems, where human experts are involved in the decision-making process, can help prevent biased outcomes and ensure fair decisions. Human oversight can act as a safeguard against unintended bias in AI systems.
8. Regular Bias Audits
Conducting regular bias audits of AI systems is essential for identifying and addressing bias issues. Bias audits involve systematically evaluating AI models for bias, fairness, and equity. By conducting regular audits, organizations can proactively detect and mitigate bias in AI systems, ensuring that they operate in a fair and unbiased manner.
9. Continuous Learning and Improvement
Emphasizing continuous learning and improvement is crucial for mitigating bias in AI systems. Organizations should foster a culture of learning and adaptation, where feedback and insights from stakeholders are used to improve AI models. By continuously refining and updating AI systems, organizations can address bias issues and enhance fairness over time.
10. Ethical Guidelines and Standards
Adhering to ethical guidelines and standards can help guide the development and deployment of AI systems in a responsible and ethical manner. Organizations should follow ethical principles such as fairness, transparency, accountability, and privacy when designing AI systems. By aligning with ethical guidelines, organizations can mitigate bias and ensure that AI systems benefit society as a whole.
What's Your Reaction?
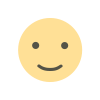
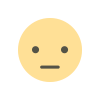
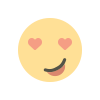
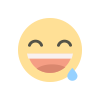
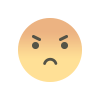
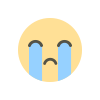
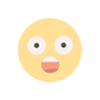