Predictive Analytics
Unlock the power of data with Predictive Analytics. Anticipate future trends, behavior, and outcomes to make informed business decisions.
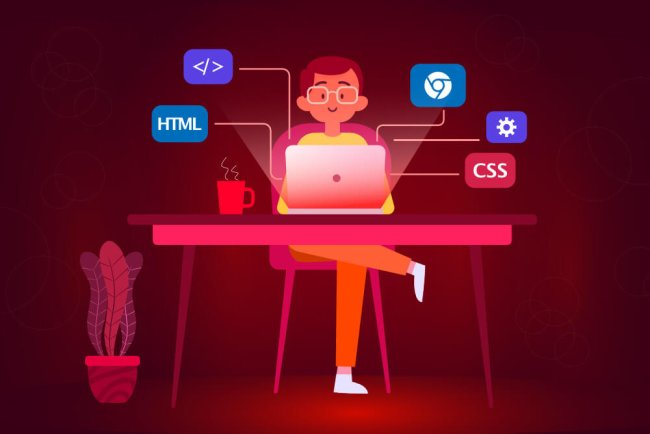
Predictive Analytics
Predictive analytics is the practice of using data, statistical algorithms, and machine learning techniques to identify the likelihood of future outcomes based on historical data. It involves extracting information from existing data sets to determine patterns and predict future trends and outcomes.
Key Components of Predictive Analytics
There are several key components of predictive analytics:
- Data Collection: The first step in predictive analytics is to gather relevant data from various sources. This data can include structured data from databases, as well as unstructured data from sources such as social media, sensors, and text documents.
- Data Analysis: Once the data is collected, it needs to be cleaned and prepared for analysis. This involves removing any inconsistencies or errors in the data and transforming it into a format that can be used for modeling.
- Modeling: In this step, statistical models and machine learning algorithms are applied to the prepared data to identify patterns and relationships. These models can range from simple linear regression to complex neural networks.
- Evaluation: After the models are built, they need to be evaluated to determine their accuracy and effectiveness. This involves testing the models on new data sets to see how well they perform in predicting outcomes.
- Deployment: Once a predictive model has been validated, it can be deployed in a real-world setting to make predictions based on new data. This can involve integrating the model into existing systems or creating a standalone application.
Applications of Predictive Analytics
Predictive analytics has a wide range of applications across various industries. Some common applications include:
- Marketing: Predictive analytics can be used to identify customer segments, predict customer behavior, and personalize marketing campaigns based on individual preferences.
- Finance: In finance, predictive analytics is used for credit scoring, fraud detection, risk assessment, and stock market forecasting.
- Healthcare: Predictive analytics is used in healthcare to predict patient outcomes, identify high-risk patients, and optimize treatment plans.
- Retail: Retailers use predictive analytics to forecast demand, optimize pricing, and improve inventory management.
- Manufacturing: In manufacturing, predictive analytics is used for predictive maintenance, quality control, and supply chain optimization.
Benefits of Predictive Analytics
There are several benefits to using predictive analytics:
- Improved Decision Making: Predictive analytics provides valuable insights that can help organizations make more informed decisions and allocate resources more effectively.
- Increased Efficiency: By predicting future outcomes, organizations can streamline operations, reduce waste, and improve overall efficiency.
- Cost Savings: Predictive analytics can help organizations identify cost-saving opportunities, such as optimizing inventory levels or reducing maintenance costs.
- Enhanced Customer Experience: By predicting customer behavior and preferences, organizations can deliver more personalized and targeted experiences to their customers.
- Competitive Advantage: Organizations that effectively leverage predictive analytics can gain a competitive edge by anticipating market trends and staying ahead of the competition.
Challenges of Predictive Analytics
While predictive analytics offers many benefits, there are also challenges associated with its implementation:
- Data Quality: The success of predictive analytics relies heavily on the quality of the data used. Poor data quality can lead to inaccurate predictions and unreliable models.
- Data Privacy and Security: Collecting and analyzing large amounts of data raises concerns about privacy and security. Organizations need to ensure that they are compliant with data protection regulations and that sensitive information is handled appropriately.
- Model Interpretability: Complex machine learning models can be difficult to interpret, making it challenging to explain the reasoning behind predictions to stakeholders.
- Implementation Complexity: Building and deploying predictive models can be a complex and resource-intensive process, requiring specialized
What's Your Reaction?
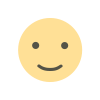
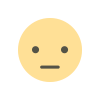
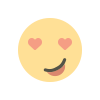
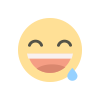
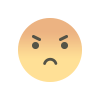
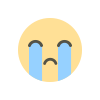
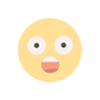