Edge AI for Personalized Recommendations
Discover how Edge AI technology is revolutionizing personalized recommendations for consumers, providing real-time insights and enhancing the shopping experience.
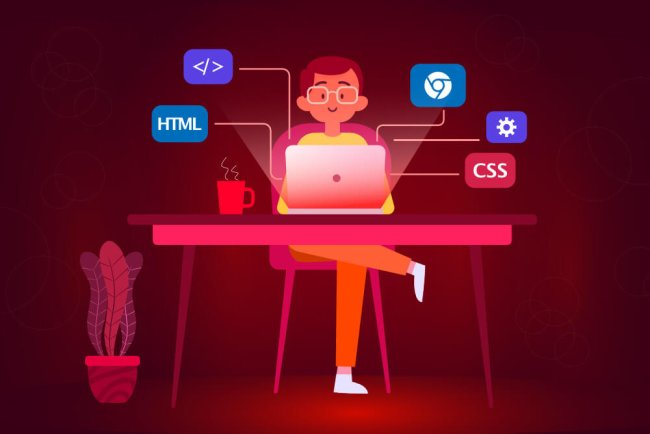
Edge AI for Personalized Recommendations
Edge AI refers to the use of artificial intelligence algorithms and models on edge devices such as smartphones, tablets, and IoT devices, enabling data processing and inference to be performed locally on the device itself. This approach offers several advantages, including reduced latency, increased privacy, and improved efficiency. One area where edge AI is making a significant impact is in personalized recommendations.
Personalized Recommendations
Personalized recommendations are a critical component of many online platforms and services, including e-commerce websites, streaming services, social media platforms, and more. By analyzing user behavior, preferences, and historical data, personalized recommendation systems can offer tailored content, products, or services to individual users, enhancing their overall experience and increasing engagement and satisfaction.
Challenges in Personalized Recommendations
Traditional recommendation systems often rely on centralized servers or cloud-based solutions to process and analyze vast amounts of data. While these systems can be effective, they also come with challenges such as latency issues, privacy concerns, and bandwidth limitations. Edge AI addresses these challenges by enabling data processing and inference to happen on the device itself, reducing the need for constant communication with a remote server.
Benefits of Edge AI for Personalized Recommendations
1. Reduced Latency: By performing data processing and inference locally on the edge device, personalized recommendations can be delivered more quickly to the user, leading to a smoother and more seamless experience.
2. Increased Privacy: Edge AI allows for user data to remain on the device and not be transmitted to a central server for processing. This helps protect user privacy and data security, as sensitive information stays within the user's control.
3. Improved Efficiency: Edge AI can optimize the use of resources on the device, such as CPU and memory, leading to more efficient processing of recommendation algorithms. This can result in faster response times and lower energy consumption.
4. Personalized Experience: By analyzing data locally on the device, edge AI can provide personalized recommendations in real-time based on the user's behavior and preferences. This leads to a more tailored and relevant user experience.
Edge AI Techniques for Personalized Recommendations
There are several edge AI techniques that can be used to enhance personalized recommendation systems:
1. Federated Learning: Federated learning enables edge devices to collaboratively train a shared machine learning model without exchanging raw data. This approach is particularly useful for personalized recommendations as it allows for model training to happen on the device while preserving user privacy.
2. On-Device Inference: By performing inference on the edge device, personalized recommendations can be generated locally without the need for a constant connection to a central server. This enables real-time recommendations and reduces latency.
3. Edge-based Collaborative Filtering: Collaborative filtering is a common technique used in recommendation systems to analyze user behavior and preferences. By implementing collaborative filtering algorithms on edge devices, personalized recommendations can be generated based on local user data.
Use Cases of Edge AI for Personalized Recommendations
1. Retail: In the retail industry, edge AI can be used to provide personalized product recommendations to shoppers in real-time based on their browsing history and preferences. This can lead to increased sales and customer satisfaction.
2. Streaming Services: Edge AI can enhance personalized recommendations on streaming platforms by analyzing user viewing habits and preferences locally on the device. This can help users discover new content tailored to their interests.
3. Social Media: Social media platforms can leverage edge AI to offer personalized content recommendations to users based on their interactions, likes, and interests. This can increase user engagement and retention on the platform.
Conclusion
Edge AI is revolutionizing personalized recommendations by enabling data processing and inference to happen on edge devices, leading to reduced latency, increased privacy, improved efficiency, and a more personalized user experience. By leveraging edge AI techniques such as federated learning, on-device inference, and edge-based collaborative filtering, online platforms and services can deliver tailored content and recommendations to users in real-time, enhancing engagement and satisfaction.
As edge AI continues to evolve and become more widespread
What's Your Reaction?
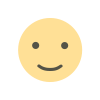
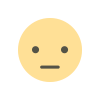
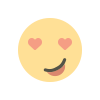
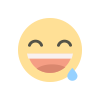
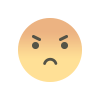
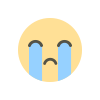
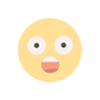