Edge AI for Surveillance
Discover how Edge AI technology is revolutionizing surveillance systems by processing data at the edge for real-time analysis and improved security.
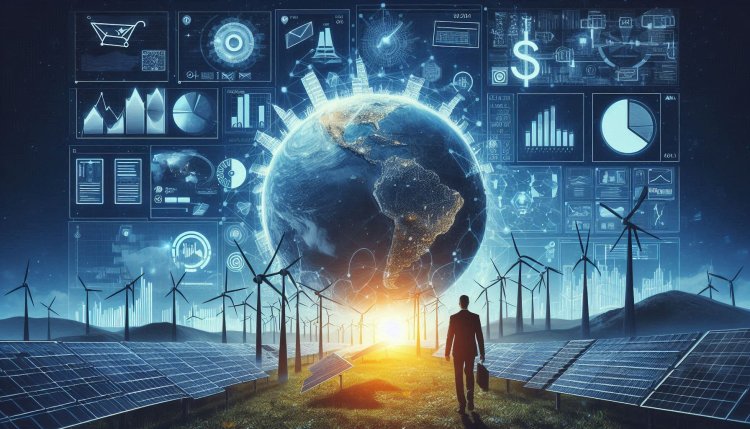
Edge AI for Surveillance
Edge AI, a combination of edge computing and artificial intelligence (AI), is revolutionizing the way surveillance systems operate. By moving the processing and analysis of data closer to the source - at the edge of the network - edge AI for surveillance offers real-time insights, reduced latency, improved privacy, and cost efficiency.
Benefits of Edge AI for Surveillance:
- Real-time Analysis: Edge AI enables surveillance systems to process and analyze data on the spot, providing real-time insights into security threats and incidents.
- Reduced Latency: By processing data locally at the edge, edge AI minimizes the delay in transmitting data to the cloud for analysis, resulting in faster response times.
- Improved Privacy: Edge AI allows for data to be processed locally without the need to send sensitive information to the cloud, enhancing privacy and security.
- Cost Efficiency: Edge AI reduces the need for continuous data transmission to the cloud, saving bandwidth costs and minimizing reliance on cloud computing resources.
Applications of Edge AI in Surveillance:
Edge AI is being applied in various surveillance scenarios to enhance security, improve monitoring capabilities, and streamline operations. Some key applications include:
- Facial Recognition: Edge AI enables real-time facial recognition for identifying individuals in a crowd, tracking persons of interest, and enhancing access control systems.
- Object Detection: Surveillance cameras equipped with edge AI can detect and classify objects, such as vehicles, weapons, or abandoned bags, to alert security personnel of potential threats.
- Anomaly Detection: Edge AI can analyze video feeds to detect unusual behaviors, such as loitering, trespassing, or sudden movements, triggering alerts for immediate action.
- Smart Monitoring: By integrating edge AI algorithms, surveillance systems can automatically monitor and analyze video streams to identify specific events or patterns, improving overall situational awareness.
Challenges and Considerations:
While edge AI offers numerous advantages for surveillance applications, there are also challenges and considerations that need to be addressed:
- Processing Power: Edge devices may have limited computing resources, requiring efficient algorithms and optimization techniques to perform AI tasks effectively.
- Data Security: Protecting data at the edge is crucial to prevent unauthorized access or tampering, necessitating robust encryption and security protocols.
- Scalability: Deploying edge AI solutions across a large surveillance network may pose scalability challenges in terms of managing multiple devices and ensuring synchronization.
- Interoperability: Ensuring compatibility and seamless integration between different edge AI devices and surveillance systems is essential for a cohesive and unified security infrastructure.
Future Trends and Innovations:
The field of edge AI for surveillance is continually evolving, driven by technological advancements and industry demands. Some emerging trends and innovations include:
- Edge-to-Cloud Collaboration: Hybrid models that combine edge processing with cloud-based analytics are being developed to leverage the strengths of both approaches for enhanced surveillance capabilities.
- Edge AI Chips: Specialized hardware chips optimized for AI inference at the edge are being designed to accelerate processing speed and efficiency for surveillance applications.
- Privacy-Preserving AI: Techniques like federated learning and differential privacy are being explored to enable AI models to learn from decentralized data sources while preserving individual privacy in surveillance settings.
- Autonomous Surveillance: AI-powered surveillance systems capable of autonomous decision-making and adaptive responses are being developed to enhance situational awareness and streamline security operations.
What's Your Reaction?
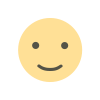
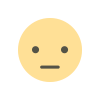
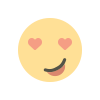
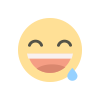
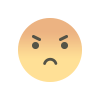
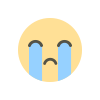
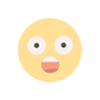