Edge AI for Recommendations
Enhance customer experience with Edge AI for Recommendations. Boost sales and engagement with personalized suggestions in real-time.
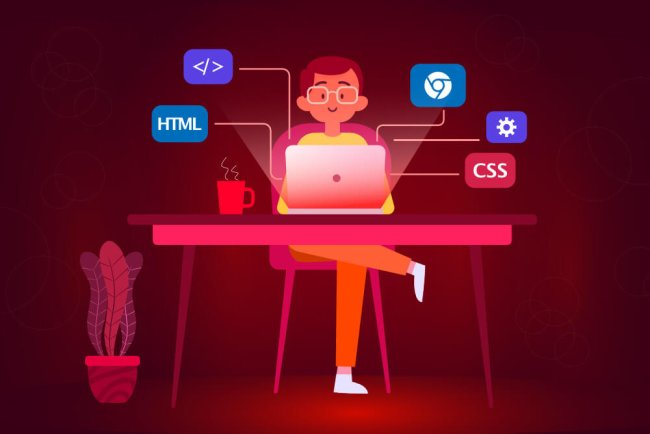
Edge AI for Recommendations
Edge AI refers to the deployment of artificial intelligence algorithms and models on edge devices such as smartphones, IoT devices, and other endpoints, rather than relying on centralized cloud servers. This approach brings several advantages, including reduced latency, increased privacy, and improved efficiency. When it comes to recommendation systems, edge AI can play a crucial role in delivering personalized content and products to users in real-time.
Challenges in Traditional Recommendation Systems
Traditional recommendation systems often rely on centralized servers to process user data and generate recommendations. This approach can lead to latency issues, especially when dealing with large datasets or when the server is located far from the end user. Additionally, concerns about data privacy and security arise when sensitive user information is transmitted to and stored on remote servers.
Benefits of Edge AI for Recommendations
By leveraging edge AI for recommendations, businesses can overcome these challenges and offer a more seamless and personalized user experience. Some key benefits of using edge AI in recommendation systems include:
- Low Latency: Edge AI enables real-time processing of user data on the device itself, reducing the time it takes to generate recommendations and deliver them to the user.
- Privacy Protection: With edge AI, user data remains on the device and is not transmitted to external servers, enhancing data privacy and security.
- Improved Efficiency: By offloading computation to edge devices, businesses can reduce the load on centralized servers and optimize resource usage.
- Personalization: Edge AI algorithms can analyze user behavior and preferences locally, allowing for more accurate and personalized recommendations without relying on a constant internet connection.
Use Cases of Edge AI for Recommendations
Edge AI can be applied to various recommendation systems across different industries, including:
- Retail: Retailers can use edge AI to provide personalized product recommendations to shoppers in real-time based on their browsing history, location, and purchase behavior.
- Streaming Services: Video streaming platforms can leverage edge AI to suggest content to users based on their viewing habits and preferences, without delays caused by server-side processing.
- Healthcare: Healthcare providers can utilize edge AI to recommend personalized treatment plans or wellness tips to patients based on their health data collected from wearable devices.
- Smart Home Devices: Smart home devices equipped with edge AI can offer customized suggestions for energy savings, security measures, and home automation based on user preferences and usage patterns.
Implementation of Edge AI for Recommendations
The implementation of edge AI for recommendations involves the following steps:
- Data Collection: User data is collected and stored locally on the edge device, ensuring data privacy and reducing the need for constant internet connectivity.
- Model Training: AI models are trained on the device using local data or pre-trained models, allowing for personalized recommendations without relying on cloud-based servers.
- Inference: The trained model makes recommendations based on real-time user interactions or input, providing instant feedback to the user without latency.
- Feedback Loop: User feedback is integrated back into the model to continuously improve the recommendation system and enhance personalization over time.
Challenges and Considerations
While edge AI offers many advantages for recommendation systems, there are some challenges and considerations to keep in mind:
- Resource Constraints: Edge devices may have limited computing power and memory, which can affect the performance of AI algorithms and the quality of recommendations.
- Data Security: Protecting user data on edge devices is crucial to prevent privacy breaches and unauthorized access to sensitive information.
- Model Updates: Updating AI models on edge devices can be challenging, as it requires efficient deployment mechanisms and version control to ensure consistency across devices.
What's Your Reaction?
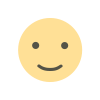
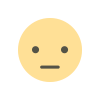
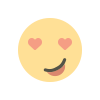
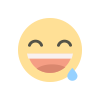
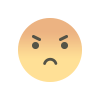
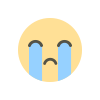
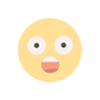