Edge AI for Human Activity Recognition
Discover how Edge AI technology is transforming human activity recognition with real-time processing for improved efficiency and accuracy. Learn more now!
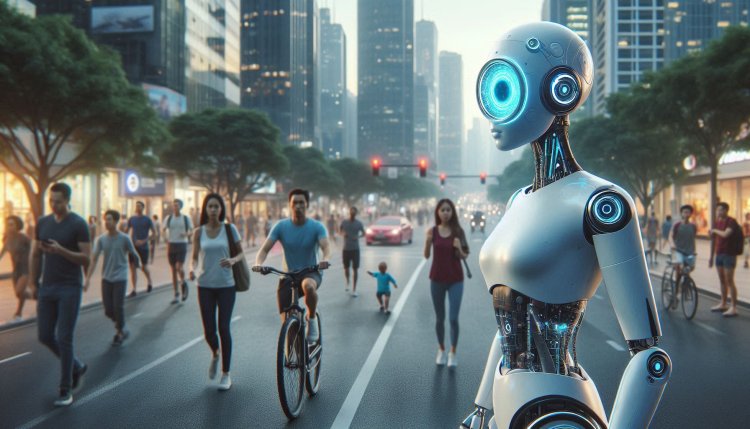
Edge AI for Human Activity Recognition
Edge AI refers to artificial intelligence algorithms and models that are deployed on edge devices such as smartphones, wearables, cameras, and IoT devices. Human Activity Recognition (HAR) is the task of identifying and classifying human activities based on sensor data such as accelerometer and gyroscope readings. By combining Edge AI with HAR, real-time activity recognition can be achieved on the edge devices themselves, without the need for constant internet connectivity or cloud resources.
Importance of Edge AI for HAR
Traditional HAR systems rely on sending raw sensor data to a centralized server for processing and classification. This approach has limitations such as latency, privacy concerns, and dependency on network connectivity. Edge AI addresses these challenges by enabling the deployment of lightweight machine learning models directly on the edge devices.
By running HAR models on edge devices, data processing and classification can be done locally, reducing the need for constant network communication. This not only improves the speed and efficiency of activity recognition but also enhances user privacy by keeping sensitive data on the device.
How Edge AI works for HAR
Edge AI for HAR typically involves the following steps:
- Data Collection: Sensor data such as accelerometer and gyroscope readings are collected by the edge device.
- Preprocessing: The raw sensor data is preprocessed to extract relevant features and normalize the data for model input.
- Model Inference: A pre-trained machine learning model for HAR is deployed on the edge device. The model takes the preprocessed sensor data as input and predicts the activity being performed.
- Feedback: The predicted activity label is sent to the user or application for feedback or further action.
Benefits of Edge AI for HAR
There are several benefits to using Edge AI for Human Activity Recognition:
- Real-time Processing: Edge AI enables real-time processing and classification of human activities, allowing for immediate feedback and response.
- Low Latency: By processing data locally on the edge device, latency is minimized compared to cloud-based solutions.
- Privacy Preservation: Edge AI keeps sensitive sensor data on the device, reducing the risk of data breaches or privacy violations.
- Offline Operation: Edge AI models can operate offline, without the need for constant internet connectivity, making them ideal for scenarios with limited network access.
- Energy Efficiency: Running AI models on edge devices consumes less power compared to transmitting data to a remote server for processing, leading to energy savings.
Challenges and Considerations
While Edge AI offers many advantages for Human Activity Recognition, there are also challenges and considerations to keep in mind:
- Device Constraints: Edge devices may have limited computational resources, memory, and battery life, which can impact the performance of AI models.
- Model Size and Complexity: Developing lightweight models that can run efficiently on edge devices while maintaining high accuracy is a key challenge.
- Data Security: Ensuring the security of sensitive sensor data stored and processed on edge devices is crucial to prevent unauthorized access.
- Model Updates: Updating AI models on edge devices can be challenging, especially for devices with limited connectivity or storage capacity.
Applications of Edge AI for HAR
Edge AI for Human Activity Recognition has a wide range of applications across various industries and domains:
- Healthcare: Monitoring patient activities for elderly care, fall detection, and rehabilitation.
- Fitness and Sports: Tracking and analyzing physical activities for fitness training and performance optimization.
- Smart Homes and IoT: Automating home appliances based on human activities and gestures.
- Industrial Safety: Ensuring worker safety by detecting and alerting to hazardous activities in industrial settings.
What's Your Reaction?
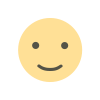
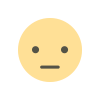
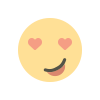
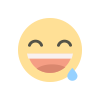
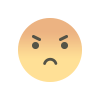
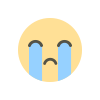
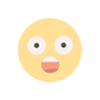