Computer Vision innovations
Discover the latest Computer Vision innovations transforming industries with advanced image recognition and analysis technology. Stay ahead with AI advancements.
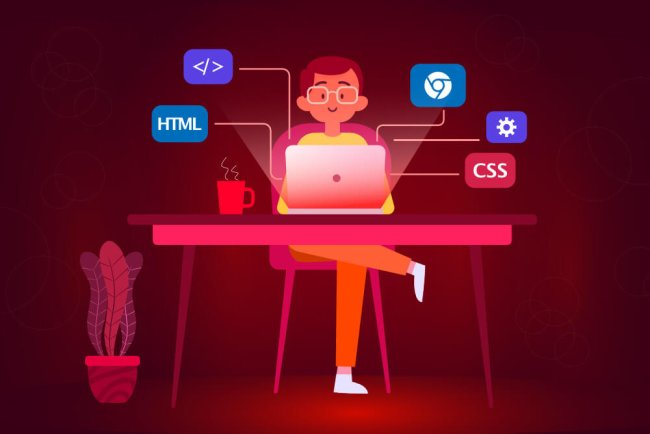
Computer Vision Innovations
Computer vision is a field of artificial intelligence that enables machines to interpret and understand the visual world. It has seen significant advancements in recent years, leading to a wide range of innovative applications across various industries. Here are some key computer vision innovations:
1. Object Detection and Recognition
Object detection and recognition are fundamental tasks in computer vision. Innovations in this area have led to highly accurate and efficient algorithms that can detect and classify objects in images and videos. These advancements have paved the way for applications such as facial recognition, autonomous driving, and image search.
2. Image Segmentation
Image segmentation is the process of partitioning an image into multiple segments or regions. Recent innovations in image segmentation algorithms have improved the accuracy and speed of segmenting objects in complex scenes. This technology is used in medical imaging, autonomous robotics, and video editing applications.
3. 3D Reconstruction
3D reconstruction is the process of creating a three-dimensional model of an object or scene from a set of 2D images. Advances in computer vision techniques have made it possible to reconstruct detailed 3D models with high accuracy and realism. This technology is used in virtual reality, augmented reality, and architectural design.
4. Image Super-Resolution
Image super-resolution is the process of enhancing the resolution and quality of low-resolution images. Recent innovations in this area have led to algorithms that can generate high-quality, detailed images from low-resolution inputs. Image super-resolution is used in medical imaging, satellite imaging, and video upscaling.
5. Video Analysis and Action Recognition
Video analysis and action recognition involve detecting and understanding actions and events in videos. Advances in computer vision have led to algorithms that can accurately recognize and classify various actions and activities in real-time video streams. This technology is used in surveillance, sports analytics, and human behavior understanding.
6. Deep Learning and Convolutional Neural Networks
Deep learning and convolutional neural networks (CNNs) have revolutionized computer vision by enabling the training of highly complex and accurate models. Deep learning algorithms, particularly CNNs, have achieved remarkable results in image classification, object detection, and image generation tasks. These techniques have been widely adopted in various computer vision applications.
7. Image Captioning and Visual Question Answering
Image captioning and visual question answering are innovative applications of computer vision that involve generating natural language descriptions of images and answering questions based on visual inputs. These technologies combine computer vision with natural language processing to enable machines to understand and communicate about visual content.
8. Transfer Learning and Pre-trained Models
Transfer learning and pre-trained models have become popular techniques in computer vision for leveraging existing knowledge and models to solve new tasks. By fine-tuning pre-trained models on specific datasets, researchers and developers can achieve high performance on a wide range of computer vision tasks with limited data and computational resources.
9. Real-time Object Tracking
Real-time object tracking is a critical component of many computer vision applications, such as surveillance, robotics, and augmented reality. Innovations in object tracking algorithms have enabled real-time and accurate tracking of objects across video frames, even in complex and dynamic environments.
10. Image Generation and Style Transfer
Image generation and style transfer technologies involve generating new images or transferring styles from one image to another. These innovations have led to the development of creative and artistic applications in computer vision, such as image synthesis, style transfer filters, and deep art generation.
11. Semantic Segmentation and Scene Understanding
Semantic segmentation and scene understanding are advanced computer vision tasks that involve assigning semantic labels to different regions in an image and understanding the overall context of a scene. Innovations in these areas have improved the ability of machines to interpret and reason about visual content, leading to more sophisticated applications in autonomous systems and robotics.
What's Your Reaction?
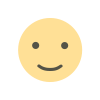
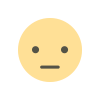
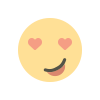
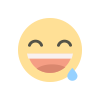
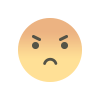
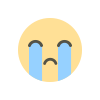
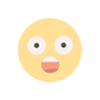