R-Squared (R^2) Score
The R-squared (R^2) score is a statistical measure that represents the proportion of the variance in the dependent variable that is predictable from the independent variable(s).
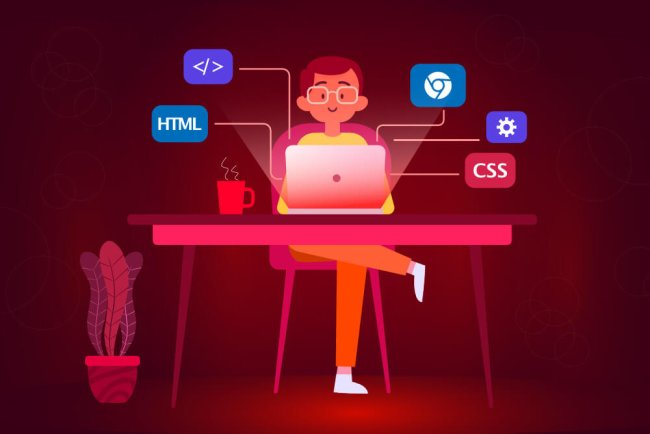
R-Squared (R^2) Score
The R-Squared (R^2) score, also known as the coefficient of determination, is a statistical measure that determines the proportion of the variance in the dependent variable that is predictable from the independent variables in a regression model.
Calculation of R-Squared Score
The R-Squared score is calculated using the following formula:
R^2 = 1 - (SS_res / SS_tot)
Where:
- R^2 is the R-Squared score
- SS_res is the sum of squared residuals (or errors) from the regression model
- SS_tot is the total sum of squares, which is the sum of squared differences between the actual values and the mean of the dependent variable
Significance of R-Squared Score
The R-Squared score is a crucial metric in regression analysis as it helps to assess how well the independent variables explain the variability in the dependent variable. A high R-Squared score indicates that a larger proportion of the variance in the dependent variable can be explained by the independent variables in the model.
On the other hand, a low R-Squared score suggests that the independent variables are not effective in predicting the dependent variable's variability. It is essential to interpret the R-Squared score in conjunction with other metrics and consider the context of the analysis to draw meaningful conclusions.
Interpretation of R-Squared Score
When interpreting the R-Squared score, it is essential to consider the following:
- An R-Squared score of 0 indicates that the independent variables do not explain any of the variability in the dependent variable.
- An R-Squared score of 1 indicates that the independent variables perfectly explain the variability in the dependent variable.
- An R-Squared score between 0 and 1 signifies the proportion of the variance in the dependent variable that can be explained by the independent variables.
- A higher R-Squared score is generally preferred as it indicates a better fit of the regression model to the data.
Limitations of R-Squared Score
While the R-Squared score is a valuable metric in regression analysis, it has certain limitations that should be taken into account:
- R-Squared does not indicate the causal relationship between independent and dependent variables. It only measures the strength of the relationship.
- R-Squared can be biased by the number of independent variables in the model. Adding more variables can artificially inflate the R-Squared score.
- R-Squared does not provide information on whether the coefficients of the independent variables are statistically significant or whether the model is overfitting the data.
Conclusion
The R-Squared (R^2) score is a fundamental measure in regression analysis that quantifies how well the independent variables explain the variability in the dependent variable. By understanding the calculation, significance, interpretation, and limitations of the R-Squared score, analysts and researchers can make informed decisions about the validity and reliability of their regression models.
It is essential to use the R-Squared score in conjunction with other evaluation metrics and domain knowledge to draw meaningful conclusions and insights from regression analyses.
For further information and practical applications of the R-Squared score in regression analysis, explore related resources, tutorials, and case studies to deepen your understanding of this critical statistical measure.
What's Your Reaction?
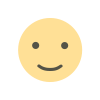
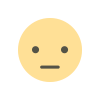
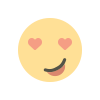
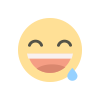
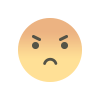
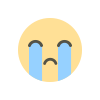
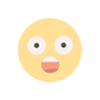