Internet of Things (IoT) and Machine Learning
Discover how the Internet of Things (IoT) and Machine Learning are revolutionizing industries and daily life, enhancing efficiency and innovation.
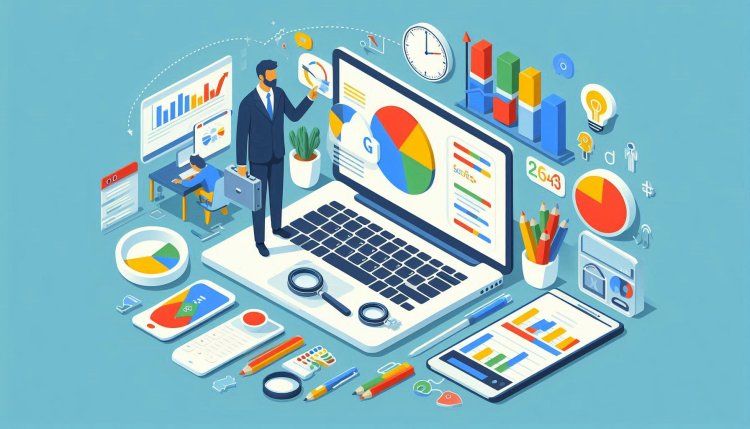
Internet of Things (IoT) and Machine Learning
The Internet of Things (IoT) and Machine Learning are two cutting-edge technologies that are revolutionizing the way we interact with the world around us. When combined, they have the potential to create powerful and intelligent systems that can automate tasks, make decisions, and improve efficiency across various industries.
Internet of Things (IoT)
The Internet of Things refers to the network of physical objects or "things" embedded with sensors, software, and other technologies that enable them to connect and exchange data with other devices and systems over the internet. These connected devices can range from everyday objects like smart home appliances and wearables to industrial equipment and infrastructure.
IoT devices collect vast amounts of data through sensors and transmit this data to centralized platforms for analysis and decision-making. This data can provide valuable insights into how these devices are being used, their performance, and any potential issues that may arise. By leveraging this data, organizations can optimize operations, improve efficiency, and deliver better services to customers.
Machine Learning
Machine Learning is a subset of artificial intelligence that focuses on developing algorithms and statistical models that enable computers to learn from and make predictions or decisions based on data. Machine Learning algorithms can analyze large datasets, identify patterns, and make intelligent decisions without explicit programming.
Machine Learning algorithms are used in a wide range of applications, such as image and speech recognition, natural language processing, recommendation systems, and predictive analytics. These algorithms can continuously learn from new data, improve their performance over time, and adapt to changing environments.
IoT and Machine Learning Integration
When IoT and Machine Learning are combined, they create a powerful synergy that enables intelligent decision-making and automation. IoT devices generate vast amounts of data, and Machine Learning algorithms can analyze this data in real-time to extract valuable insights and drive actionable outcomes.
Some key applications of integrating IoT and Machine Learning include:
- Predictive Maintenance: Machine Learning algorithms can analyze IoT sensor data to predict when equipment is likely to fail, enabling proactive maintenance and reducing downtime.
- Smart Energy Management: IoT devices can monitor energy usage in real-time, and Machine Learning algorithms can optimize energy consumption based on patterns and user behavior.
- Healthcare Monitoring: IoT wearables can collect health data, and Machine Learning models can analyze this data to provide personalized insights and early detection of health issues.
- Supply Chain Optimization: IoT sensors can track inventory and shipments, and Machine Learning algorithms can optimize logistics, predict demand, and improve supply chain efficiency.
Challenges and Considerations
While the integration of IoT and Machine Learning offers significant benefits, there are also challenges and considerations to keep in mind:
- Data Security: With the influx of data generated by IoT devices, ensuring data security and privacy is crucial to prevent unauthorized access or breaches.
- Scalability: As the number of connected devices grows, managing and scaling Machine Learning models to handle the volume of data can be a complex task.
- Interoperability: Ensuring compatibility and seamless integration between different IoT devices and Machine Learning algorithms can be a challenge, especially in heterogeneous environments.
- Ethical Considerations: The use of IoT data and Machine Learning algorithms raises ethical concerns around data ownership, transparency, bias, and accountability.
Future Trends
The convergence of IoT and Machine Learning is driving innovation and shaping the future of technology across various industries. Some emerging trends to watch out for include:
- Edge Computing: With the rise of IoT devices at the edge of networks, there is a growing need for processing data locally to reduce latency and bandwidth requirements.
- Federated Learning: This approach allows Machine Learning models to be trained across multiple decentralized devices without sharing sensitive data, enabling privacy-preserving collaborations.
What's Your Reaction?
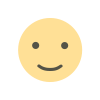
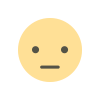
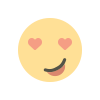
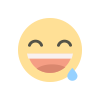
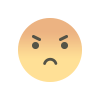
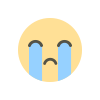
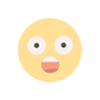