Ethical Considerations in Machine Learning
Explore the ethical implications of machine learning technology, including bias, privacy concerns, and transparency in decision-making processes.
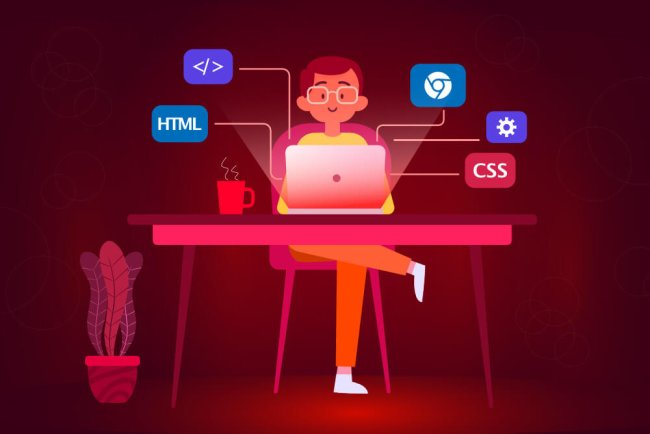
Ethical Considerations in Machine Learning
Machine learning, a subset of artificial intelligence, has the potential to revolutionize industries and improve various aspects of our lives. However, as with any powerful technology, there are ethical considerations that must be taken into account to ensure that its applications are beneficial and fair to all individuals and communities. Here are some key ethical considerations in machine learning:
1. Bias and Fairness
One of the primary ethical concerns in machine learning is bias in data and algorithms. Machine learning systems are only as good as the data they are trained on, and if the training data is biased, the algorithms will perpetuate and even amplify that bias. This can lead to discriminatory outcomes in areas such as hiring, lending, and criminal justice. It is essential to carefully examine training data, identify biases, and take steps to mitigate them to ensure fair and equitable outcomes.
2. Transparency and Accountability
Machine learning algorithms can be complex and difficult to interpret, making it challenging to understand how decisions are being made. Lack of transparency can lead to mistrust and raise concerns about accountability. It is crucial for organizations to be transparent about how machine learning systems operate, including the data used, the algorithms employed, and the decisions made. Establishing clear accountability mechanisms and providing explanations for algorithmic decisions can help build trust with users and stakeholders.
3. Privacy and Data Protection
Machine learning relies on vast amounts of data to train algorithms and make predictions. This raises concerns about privacy and data protection, especially when sensitive personal information is involved. Organizations must ensure that data is collected and used in a manner that respects individuals' privacy rights and complies with relevant regulations, such as the General Data Protection Regulation (GDPR). Implementing data anonymization techniques and adopting privacy-enhancing technologies can help mitigate privacy risks.
4. Security and Robustness
Machine learning systems are vulnerable to attacks and manipulation, which can have serious consequences, such as misinformation, fraud, and breaches of privacy. It is essential to design machine learning models with security in mind, taking measures to protect against adversarial attacks and ensure robustness against unexpected inputs. Regular security audits and testing can help identify vulnerabilities and strengthen the resilience of machine learning systems.
5. Bias in Representation
Another ethical consideration in machine learning is the lack of diversity and representation in the development and deployment of algorithms. If machine learning systems are built by homogenous teams or trained on biased datasets, they may not adequately account for the needs and perspectives of diverse populations. It is important to prioritize diversity and inclusion in the development of machine learning technologies to ensure that they are fair and inclusive for all users.
6. Ethical Decision-Making
Machine learning systems are increasingly being used to make decisions that impact individuals' lives, such as determining credit scores, predicting health outcomes, and recommending job candidates. It is crucial to consider the ethical implications of these decisions and ensure that they align with societal values and norms. Organizations should establish ethical guidelines and frameworks for the development and deployment of machine learning systems, incorporating principles such as transparency, fairness, and accountability.
7. Social Impact and Responsibility
Machine learning technologies have the potential to have far-reaching social impacts, affecting areas such as employment, education, and healthcare. Organizations developing and deploying machine learning systems have a responsibility to consider the broader societal implications of their technologies and take steps to mitigate negative consequences. Engaging with stakeholders, including affected communities and experts in ethics and social sciences, can help organizations understand and address the social impacts of machine learning.
8. Continuous Monitoring and Evaluation
Ethical considerations in machine learning are not static and evolve as technology advances and new challenges emerge. Organizations must establish mechanisms for continuous monitoring and evaluation of the ethical implications of their machine learning systems, identifying and addressing issues as they arise. Regular audits, reviews, and feedback loops can help ensure that machine learning applications remain ethical and aligned with societal values.
Conclusion
Addressing ethical considerations in machine learning is essential to ensure that the benefits of this technology are realized in a responsible and sustainable manner. By prioritizing fairness, transparency, privacy, security, diversity, and ethical decision-making, organizations can develop machine learning systems that are trustworthy, inclusive, and aligned with societal values.
What's Your Reaction?
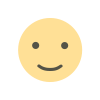
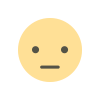
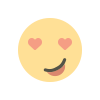
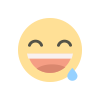
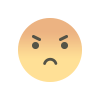
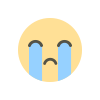
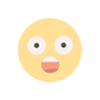