Edge AI Hardware
Edge AI hardware accelerates machine learning tasks on devices, reducing latency and enhancing privacy. Discover the latest advancements in edge AI technology.
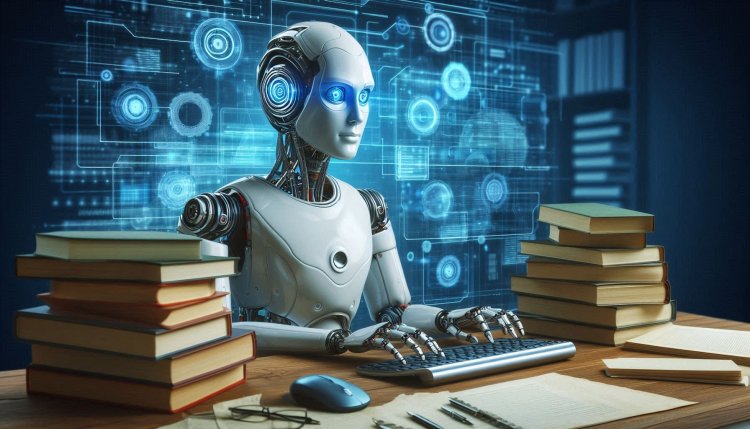
Edge AI Hardware
Edge AI hardware refers to specialized hardware components that are designed to run artificial intelligence algorithms and models directly on edge devices, such as smartphones, cameras, drones, and IoT devices. By processing AI tasks locally on the device itself, edge AI hardware can provide faster response times, increased privacy, and reduced reliance on cloud computing resources.
Key Features of Edge AI Hardware
Edge AI hardware typically includes the following key features:
- Low Power Consumption: Edge AI hardware is designed to be power-efficient to operate on battery-powered devices for extended periods without draining the battery quickly.
- High Performance: Despite being power-efficient, edge AI hardware is capable of delivering high performance for running complex AI models and algorithms in real-time.
- On-Device Processing: Edge AI hardware enables on-device processing of AI tasks, reducing the need to send data to remote servers for processing, which can lead to lower latency and improved privacy.
- Compact Form Factor: Edge AI hardware is often designed to be compact and lightweight to fit into smaller devices without compromising on performance.
- Scalability: Edge AI hardware can be scalable to support a variety of AI workloads, from simple image recognition tasks to more complex natural language processing.
Types of Edge AI Hardware
There are several types of edge AI hardware available in the market, each designed for specific use cases and performance requirements. Some common types include:
- System-on-Chip (SoC): SoC devices integrate the CPU, GPU, and other components onto a single chip, offering a compact and power-efficient solution for edge AI processing.
- Field-Programmable Gate Array (FPGA): FPGAs are programmable hardware devices that can be customized for specific AI workloads, providing flexibility and high performance for edge computing applications.
- Neural Processing Unit (NPU): NPUs are specialized hardware accelerators designed specifically for running neural network models efficiently, making them ideal for AI inference tasks at the edge.
- Graphics Processing Unit (GPU): GPUs are commonly used for accelerating AI workloads, offering parallel processing capabilities that can speed up neural network computations on edge devices.
- Tensor Processing Unit (TPU): TPUs are Google's custom-designed hardware accelerators optimized for machine learning workloads, providing high performance and energy efficiency for edge AI applications.
Applications of Edge AI Hardware
Edge AI hardware is being used in a wide range of applications across various industries, including:
- Smart Home Devices: Edge AI hardware enables smart home devices, such as security cameras and voice assistants, to process data locally without relying on cloud services, improving response times and privacy.
- Autonomous Vehicles: Edge AI hardware plays a crucial role in enabling real-time decision-making for autonomous vehicles by processing sensor data and running AI algorithms onboard the vehicle.
- Healthcare: Edge AI hardware is used in wearable devices and medical equipment to monitor patient health, analyze data, and provide personalized healthcare solutions without the need for constant internet connectivity.
- Retail: Edge AI hardware powers intelligent retail solutions, such as smart checkout systems, personalized recommendations, and inventory management, improving customer experience and operational efficiency.
- Industrial IoT: Edge AI hardware enables predictive maintenance, quality control, and process optimization in industrial IoT applications by processing sensor data locally and providing real-time insights for decision-making.
What's Your Reaction?
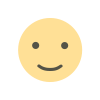
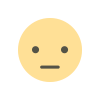
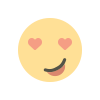
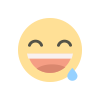
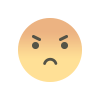
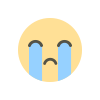
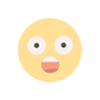