Edge AI for Supply Chain Visibility
Unlock real-time insights and streamline operations with Edge AI for Supply Chain Visibility. Enhance efficiency and visibility in your supply chain process.
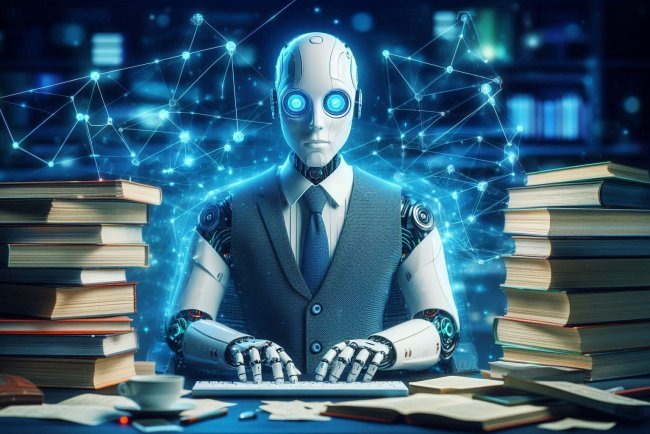
Edge AI for Supply Chain Visibility
Supply chain visibility is crucial for businesses to effectively manage their operations and meet customer demands. With the rise of edge AI technology, companies now have the ability to enhance their supply chain visibility like never before. Edge AI refers to artificial intelligence algorithms that are processed locally on a device, such as a sensor or a gateway, rather than relying on cloud-based computing. This enables real-time data processing and analysis at the edge of the network, leading to faster decision-making and improved efficiency.
Benefits of Edge AI for Supply Chain Visibility
Implementing edge AI in supply chain management offers several key benefits:
- Real-time Data Processing: Edge AI allows for real-time processing of data collected from IoT devices and sensors in the supply chain. This enables companies to quickly analyze and act on critical information, leading to faster response times and improved decision-making.
- Reduced Latency: By processing data at the edge of the network, companies can significantly reduce latency in their supply chain operations. This is particularly important for time-sensitive processes, such as inventory management and order fulfillment.
- Improved Predictive Analytics: Edge AI enables companies to leverage advanced analytics and machine learning models to predict supply chain disruptions and proactively address potential issues. This helps businesses optimize their operations and minimize risks.
- Enhanced Security and Privacy: Processing data locally at the edge enhances security and privacy by reducing the need to transmit sensitive information to the cloud. This helps protect valuable supply chain data from potential cyber threats and breaches.
- Cost Efficiency: Edge AI can help reduce costs associated with cloud computing and data storage by processing and analyzing data locally. This can lead to significant cost savings for companies managing large-scale supply chain operations.
Use Cases of Edge AI in Supply Chain Visibility
There are several use cases where edge AI can transform supply chain visibility:
- Inventory Management: Edge AI can be used to monitor and track inventory levels in real-time, enabling companies to optimize their stock levels, reduce carrying costs, and prevent stockouts.
- Asset Tracking: By deploying edge AI-enabled sensors and devices, businesses can track the location and condition of assets throughout the supply chain, improving overall visibility and asset utilization.
- Predictive Maintenance: Edge AI can help predict equipment failures and maintenance needs in advance, allowing companies to schedule maintenance activities proactively and avoid costly downtime.
- Risk Management: Edge AI can analyze various data points in the supply chain to identify potential risks, such as disruptions in transportation routes or supplier delays, and help companies develop contingency plans to mitigate these risks.
- Quality Control: By deploying edge AI for real-time quality control inspections, companies can ensure that products meet quality standards before they are shipped to customers, reducing the likelihood of returns and improving customer satisfaction.
Challenges and Considerations
While edge AI offers significant advantages for supply chain visibility, there are also challenges and considerations to keep in mind:
- Integration Complexity: Integrating edge AI technologies into existing supply chain systems can be complex and require specialized expertise. Companies need to carefully plan and execute their integration strategies to ensure seamless operation.
- Data Security: As data is processed and analyzed at the edge, companies need to implement robust security measures to protect sensitive information from cyber threats. This includes encryption, authentication protocols, and regular security audits.
- Scalability: Companies must consider the scalability of their edge AI solutions to accommodate growing supply chain operations and data volumes. Scalability challenges may arise if the infrastructure is not designed to handle increased demand.
- Training and Maintenance: Edge AI models require regular training and maintenance to ensure optimal performance. Companies need to allocate resources for training data collection, model updates, and monitoring to keep their AI systems accurate and reliable.
What's Your Reaction?
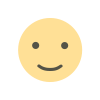
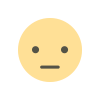
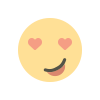
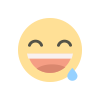
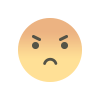
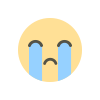
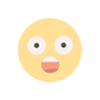