Edge AI for Risk Management
Discover how Edge AI is transforming risk management by enabling real-time data analysis and decision-making at the edge of the network.
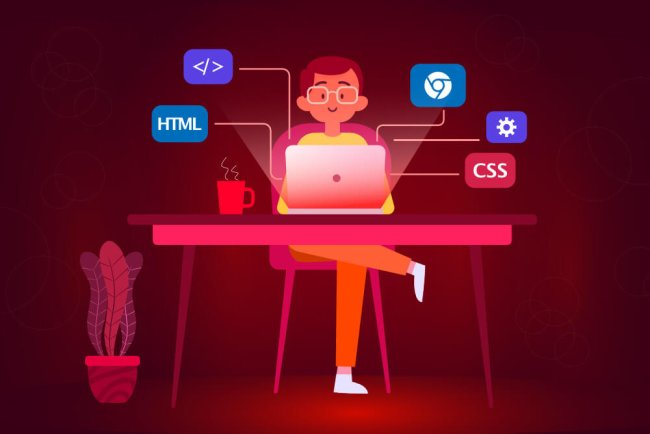
Edge AI for Risk Management
Risk management is a critical aspect of business operations, encompassing the identification, assessment, and prioritization of risks followed by coordinated and economical application of resources to minimize, monitor, and control the probability and impact of unfortunate events.
Advancements in technology have enabled the integration of Artificial Intelligence (AI) into various business processes, including risk management. Edge AI, a subset of AI that processes data locally on a hardware device, is gaining prominence in the domain of risk management due to its ability to analyze data in real-time and provide actionable insights at the edge of the network.
Benefits of Edge AI for Risk Management
1. Real-time Risk Assessment: Edge AI enables real-time analysis of data at the edge of the network, allowing for immediate risk assessment and response. This capability is particularly valuable in scenarios where quick decision-making is essential to mitigate potential risks.
2. Improved Data Privacy and Security: By processing data locally on the edge device, Edge AI reduces the need to transmit sensitive information to centralized servers for analysis. This helps in enhancing data privacy and security, especially in industries where data protection is a top priority.
3. Enhanced Predictive Analytics: Edge AI algorithms can leverage historical data and real-time inputs to predict potential risks and trends. This predictive analytics capability enables organizations to proactively address emerging risks before they escalate into significant issues.
4. Scalability and Flexibility: Edge AI solutions can be easily deployed across a distributed network of edge devices, providing scalability and flexibility in risk management processes. This decentralized approach ensures that risk assessment and mitigation can be performed efficiently across various locations.
Applications of Edge AI in Risk Management
1. Fraud Detection: Edge AI can be utilized for real-time fraud detection in financial transactions by analyzing transaction data at the point of sale or online payment gateway. By detecting anomalies and patterns indicative of fraudulent activities, organizations can prevent financial losses and protect customer accounts.
2. Cybersecurity: Edge AI plays a crucial role in enhancing cybersecurity measures by monitoring network traffic, identifying potential threats, and initiating immediate responses to mitigate cyber risks. By detecting and blocking malicious activities at the edge of the network, organizations can strengthen their defense mechanisms against cyber attacks.
3. Supply Chain Management: Edge AI can optimize supply chain operations by analyzing data from IoT sensors, RFID tags, and other connected devices at various points along the supply chain. By identifying potential disruptions or delays in advance, organizations can take proactive measures to mitigate risks and ensure smooth operations.
4. Asset Management: Edge AI can be used for real-time monitoring of assets, such as equipment, vehicles, and inventory, to detect anomalies, predict maintenance needs, and prevent potential breakdowns. By leveraging predictive analytics at the edge, organizations can optimize asset utilization and minimize downtime.
Challenges and Considerations
While Edge AI offers numerous benefits for risk management, there are also challenges and considerations that organizations need to address:
1. Data Quality and Integration: Ensuring the quality and consistency of data inputs from edge devices is crucial for the accuracy of AI algorithms. Organizations must establish robust data integration processes and mechanisms to handle diverse data sources effectively.
2. Edge Device Capabilities: The processing power and storage capacity of edge devices may vary, impacting the performance of AI algorithms deployed at the edge. Organizations need to assess the capabilities of edge devices and optimize algorithms accordingly for efficient processing.
3. Security and Privacy Concerns: As data is processed locally on edge devices, ensuring the security and privacy of sensitive information is paramount. Organizations must implement robust security measures to protect data at the edge and during transmission to centralized systems.
4. Regulatory Compliance: Organizations operating in regulated industries must adhere to compliance requirements related to data handling, privacy, and security. Deploying Edge AI solutions for risk management necessitates compliance with relevant regulations to avoid legal and financial implications.
What's Your Reaction?
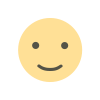
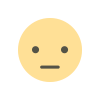
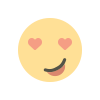
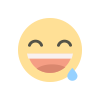
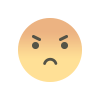
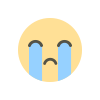
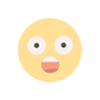