Edge AI for Plant Disease Detection
Harness the power of Edge AI for accurate and efficient plant disease detection. Improve crop health and yield with real-time monitoring and analysis.
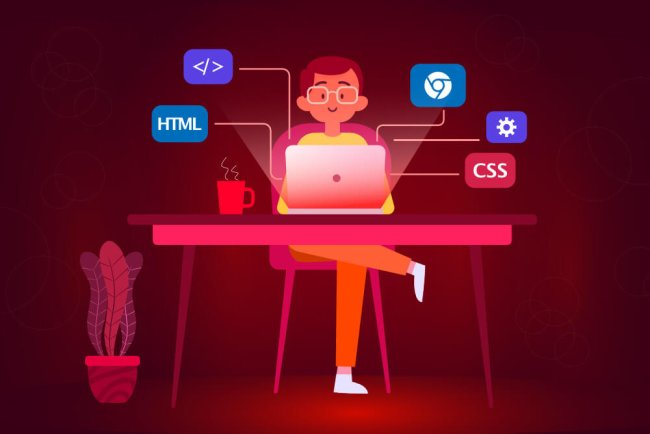
Edge AI for Plant Disease Detection
Plant diseases pose a significant threat to agricultural productivity and food security worldwide. Early detection and timely intervention are crucial for effective disease management. The integration of edge AI technology in plant disease detection systems offers a promising solution to this challenge.
What is Edge AI?
Edge AI refers to the deployment of artificial intelligence algorithms on edge devices such as smartphones, cameras, sensors, and other IoT devices, enabling them to perform AI-related tasks locally without relying on cloud computing resources. This allows for real-time processing of data at the edge of the network, reducing latency and enhancing privacy and security.
Application in Plant Disease Detection
Edge AI technology can be leveraged for the development of smart agriculture systems that enable early and accurate detection of plant diseases. By integrating AI algorithms into edge devices like drones, cameras, or sensors deployed in the field, farmers can efficiently monitor the health of their crops and detect signs of diseases in real-time.
Key Benefits of Edge AI in Plant Disease Detection:
- Real-time Detection: Edge AI enables instant processing of images or sensor data, allowing for immediate detection of disease symptoms in plants.
- Reduced Latency: By processing data locally on edge devices, the time taken to analyze and respond to disease outbreaks is significantly reduced.
- Cost-effective: Edge AI eliminates the need for continuous connectivity to cloud servers, reducing data transfer costs and operational expenses.
- Privacy and Security: Data processed on edge devices remains on-premises, enhancing privacy and security by minimizing the risk of data breaches.
- Scalability: Edge AI systems can be easily scaled to accommodate large farming operations, providing a flexible and adaptable solution for agriculture management.
Implementation of Edge AI for Plant Disease Detection
The implementation of edge AI for plant disease detection typically involves the following steps:
- Data Collection: Images or sensor data are collected from the field using drones, cameras, or IoT devices.
- Data Pre-processing: The collected data is pre-processed to enhance image quality, remove noise, and prepare it for analysis.
- Feature Extraction: Relevant features are extracted from the pre-processed data to identify disease symptoms in plants.
- Model Training: AI algorithms are trained on the extracted features to learn patterns associated with different plant diseases.
- Inference: The trained model is deployed on edge devices to perform real-time inference, detecting diseases in plants based on the input data.
- Alert Generation: When a disease is detected, alerts can be generated for farmers to take necessary actions such as applying pesticides or implementing control measures.
Challenges and Future Directions
While edge AI shows great promise for plant disease detection, there are several challenges that need to be addressed to realize its full potential:
- Data Quality: Ensuring the accuracy and reliability of data collected from edge devices is essential for effective disease detection.
- Model Optimization: Developing lightweight and efficient AI models that can run on resource-constrained edge devices without compromising performance.
- Interoperability: Ensuring seamless integration and communication between edge devices, cloud servers, and agricultural management systems.
- Adoption and Training: Educating farmers and agricultural stakeholders on the benefits of edge AI technology and providing training on its implementation and use.
Looking ahead, the future of plant disease detection using edge AI holds great promise for revolutionizing agriculture practices and enhancing crop yield and quality. By leveraging the power of AI at the edge of the network, farmers can make informed decisions in real-time, leading to more sustainable and efficient farming practices.
What's Your Reaction?
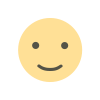
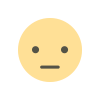
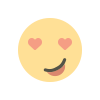
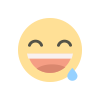
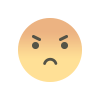
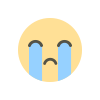
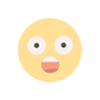