Edge AI for Event Detection
Discover how Edge AI technology is revolutionizing event detection with real-time analysis and response, enhancing security and efficiency.
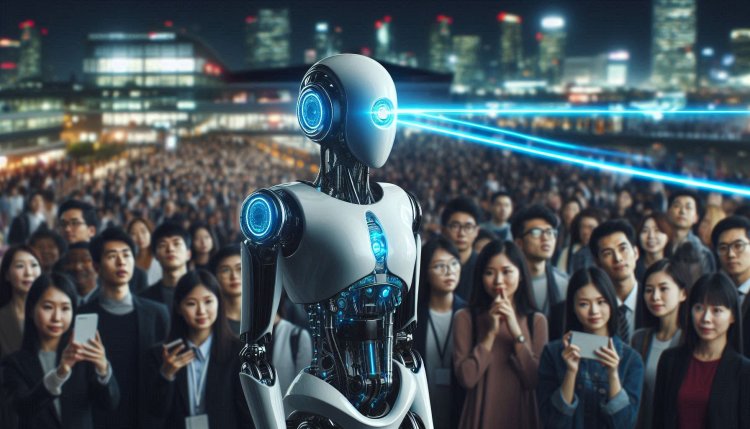
Edge AI for Event Detection
Edge AI refers to the deployment of artificial intelligence algorithms and models on edge devices, such as sensors, cameras, and other IoT devices, allowing data processing and analysis to be performed locally on the device itself, rather than relying on a centralized cloud server. This approach has gained popularity due to its ability to reduce latency, improve privacy, and increase efficiency in data processing.
Event detection is a crucial task in various applications, such as surveillance, smart cities, industrial automation, and healthcare. It involves identifying and classifying specific events or activities from a stream of data, such as video feeds or sensor readings. Edge AI for event detection leverages the power of machine learning and deep learning algorithms to enable real-time, on-device processing and detection of events without the need for constant connectivity to the cloud.
Benefits of Edge AI for Event Detection
There are several benefits to using edge AI for event detection:
- Reduced Latency: By processing data locally on the edge device, event detection can be performed in real-time, leading to faster response times.
- Improved Privacy: Since data is processed on the device itself, sensitive information does not need to be transmitted to a remote server, enhancing privacy and security.
- Efficient Use of Resources: Edge AI enables devices to filter and analyze data locally, reducing the amount of data that needs to be sent to the cloud for processing, thereby optimizing bandwidth and storage usage.
- Increased Reliability: Edge AI systems can continue to function even in the absence of a network connection, ensuring uninterrupted event detection capabilities.
Applications of Edge AI for Event Detection
Edge AI for event detection has a wide range of applications across various industries:
- Smart Cities: Edge AI can be used to detect traffic congestion, pedestrian movements, and other events to optimize city planning and resource allocation.
- Industrial Automation: In manufacturing and industrial settings, edge AI can detect equipment failures, monitor production lines, and ensure operational efficiency.
- Healthcare: Edge AI can aid in patient monitoring, fall detection, and activity recognition for elderly care and medical applications.
- Security and Surveillance: Edge AI is essential for real-time detection of security breaches, intrusions, and suspicious activities in video feeds and sensor data.
Challenges and Considerations
While edge AI for event detection offers numerous advantages, there are also challenges and considerations to be aware of:
- Resource Constraints: Edge devices typically have limited computational power and memory, which can constrain the complexity of AI models that can be deployed.
- Data Quality and Variability: The quality and variability of data collected by edge devices can affect the performance of AI algorithms, requiring robust preprocessing and filtering techniques.
- Model Training and Updates: Continuous model training and updates may be challenging on edge devices with limited connectivity and storage capabilities, necessitating efficient strategies for model optimization.
- Security and Privacy: Safeguarding sensitive data processed on edge devices from security threats and ensuring compliance with privacy regulations are critical considerations in deploying edge AI solutions.
Future Trends in Edge AI for Event Detection
As edge computing and AI technologies continue to advance, several trends are shaping the future of event detection on the edge:
- Federated Learning: Federated learning enables collaborative model training across multiple edge devices without centralized data aggregation, preserving data privacy and improving model performance.
- Hardware Acceleration: The development of specialized hardware, such as edge AI processors and accelerators, is enhancing the computational capabilities of edge devices for running complex AI algorithms efficiently.
- Edge-to-Cloud Integration: Hybrid edge-to-cloud architectures allow for seamless integration of edge AI with cloud-based services, enabling scalable and adaptive event detection solutions.
What's Your Reaction?
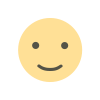
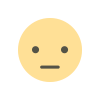
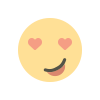
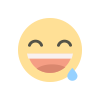
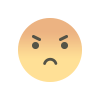
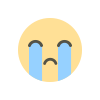
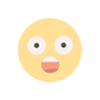