Edge AI for Emotion Recognition
Discover how Edge AI technology can accurately recognize emotions in real-time, enabling personalized experiences and improved human-computer interactions.
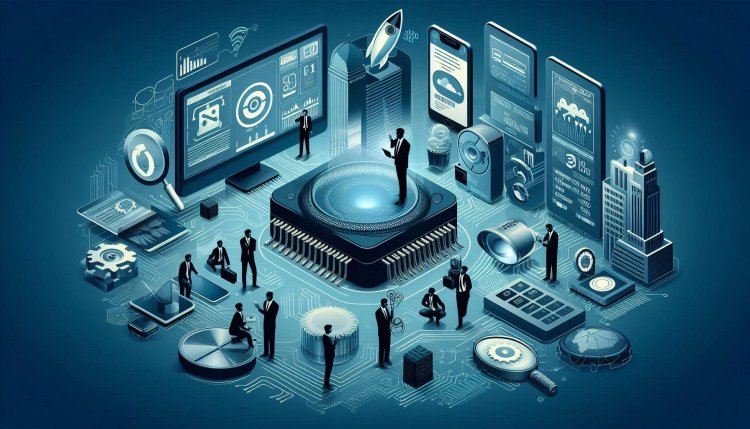
Edge AI for Emotion Recognition
Edge AI for emotion recognition is a cutting-edge technology that leverages artificial intelligence algorithms to analyze facial expressions and gestures in real-time, enabling devices to detect and interpret human emotions without relying on cloud-based processing.
How Does Edge AI for Emotion Recognition Work?
Unlike traditional approaches that require sending data to a remote server for processing, edge AI for emotion recognition performs the analysis directly on the device itself, such as a smartphone, camera, or IoT device. This allows for faster and more efficient processing, as well as enhanced privacy and security.
The technology uses deep learning models, such as convolutional neural networks (CNNs) and recurrent neural networks (RNNs), to extract features from facial expressions, body language, and speech patterns. These models are trained on large datasets of labeled emotion data to accurately recognize emotions such as happiness, sadness, anger, surprise, and more.
Once the models are deployed on the edge device, they can analyze live video streams or audio inputs in real-time to detect and classify the emotions of the user. This information can then be used to personalize user experiences, improve customer service, enhance mental health monitoring, and enable new applications in various industries.
Benefits of Edge AI for Emotion Recognition
There are several benefits to using edge AI for emotion recognition, including:
- Real-time Processing: By analyzing data locally on the device, edge AI allows for faster response times and reduced latency, making it ideal for applications that require real-time emotion detection.
- Privacy and Security: Since data is processed locally, there is no need to send sensitive information to external servers, ensuring user privacy and data security.
- Efficiency: Edge AI reduces the need for constant internet connectivity and cloud-based processing, saving bandwidth and energy consumption.
- Customization: The ability to deploy and customize emotion recognition models on edge devices allows for personalized user experiences and tailored applications.
Applications of Edge AI for Emotion Recognition
Edge AI for emotion recognition has a wide range of applications across various industries, including:
- Healthcare: In healthcare, edge AI can be used to monitor patient emotions, detect signs of depression or anxiety, and provide personalized mental health support.
- Retail: Retailers can use emotion recognition to analyze customer reactions to products, optimize store layouts, and enhance customer engagement.
- Automotive: In the automotive industry, emotion recognition can be integrated into driver monitoring systems to detect drowsiness, distraction, and other emotional states for improved safety.
- Education: Edge AI can be used in educational settings to assess student engagement, tailor learning experiences, and provide feedback based on emotional cues.
Challenges and Considerations
While edge AI for emotion recognition offers numerous benefits, there are also challenges and considerations to be aware of, including:
- Hardware Limitations: Edge devices may have limited processing power and memory, which can impact the performance of emotion recognition models.
- Data Privacy: Ensuring the privacy and security of user data is crucial, especially when processing sensitive information such as emotions.
- Model Accuracy: Training emotion recognition models to accurately detect a wide range of emotions requires large and diverse datasets, which may be challenging to obtain.
- Deployment and Maintenance: Managing and updating AI models on edge devices can be complex and require ongoing maintenance to ensure optimal performance.
Future Trends in Edge AI for Emotion Recognition
As edge AI technologies continue to evolve, there are several emerging trends to watch in the field of emotion recognition, including:
- Federated Learning: Federated learning allows edge devices to collaboratively train emotion recognition models without sharing raw data, enabling privacy-preserving and decentralized machine learning.
What's Your Reaction?
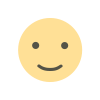
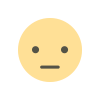
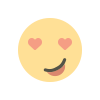
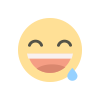
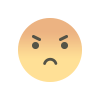
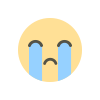
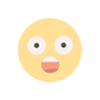