Edge AI for Crop Monitoring
Boost crop monitoring efficiency with Edge AI technology. Analyze real-time data for better decision-making. Improve yields and reduce resource usage.
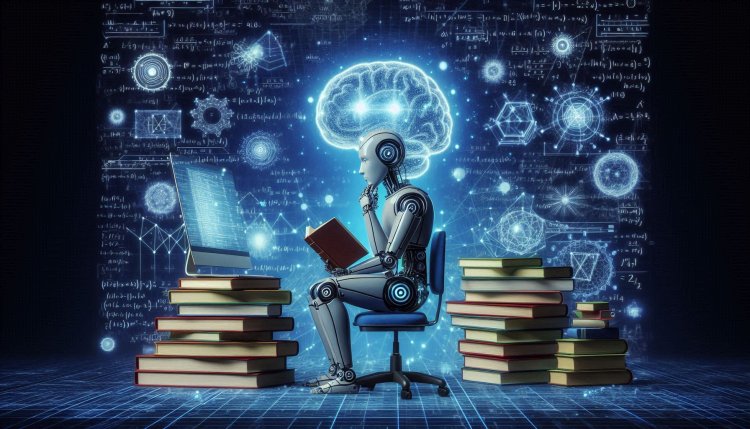
Edge AI for Crop Monitoring
Edge AI, or Edge Artificial Intelligence, refers to the use of artificial intelligence algorithms on edge computing devices, such as sensors, drones, or other IoT devices, to process data locally without needing to send it to a centralized cloud server. This approach offers benefits like faster processing, reduced latency, improved data privacy, and lower bandwidth usage. When applied to crop monitoring, Edge AI can revolutionize agriculture practices by providing real-time insights and decision-making capabilities to farmers.
Benefits of Edge AI in Crop Monitoring
There are several benefits of using Edge AI for crop monitoring:
- Real-time Monitoring: Edge AI enables real-time monitoring of crop health, soil conditions, and environmental factors. Farmers can receive instant alerts about pest infestations, diseases, or drought conditions, allowing for timely interventions to protect their crops.
- Improved Efficiency: By processing data locally on edge devices, farmers can reduce the time and resources needed to analyze large datasets. This leads to more efficient decision-making and resource allocation, ultimately increasing crop yields and profitability.
- Data Privacy: Since data is processed locally on the edge devices, farmers can maintain greater control over their sensitive agricultural data. This helps protect against data breaches and ensures the privacy and security of farm-related information.
- Lower Costs: Edge AI can help reduce costs associated with cloud computing services and data transmission. By leveraging existing edge devices and infrastructure, farmers can implement AI-powered crop monitoring solutions at a lower cost.
Applications of Edge AI in Crop Monitoring
Edge AI can be applied to various aspects of crop monitoring, including:
- Pest Detection: Edge AI algorithms can analyze images captured by drones or sensors to identify pest infestations in crops. Early detection of pests allows farmers to take targeted actions to prevent crop damage.
- Disease Identification: By processing plant images on edge devices, AI models can detect signs of diseases or nutrient deficiencies in crops. Farmers can then apply appropriate treatments to prevent the spread of diseases and ensure crop health.
- Soil Analysis: Edge AI can analyze soil samples collected by sensors to assess soil quality, nutrient levels, and moisture content. This information helps farmers optimize fertilization and irrigation practices for improved crop growth.
- Weather Forecasting: Edge AI models can process local weather data to provide accurate forecasts for specific farm locations. Farmers can use this information to make informed decisions about planting schedules, irrigation timing, and pest management strategies.
Case Study: Smart Agriculture with Edge AI
One example of the application of Edge AI in crop monitoring is the use of smart agriculture systems in vineyards. In this case study, sensors and cameras are deployed in vineyards to collect data on grapevines' health, growth, and environmental conditions. Edge AI algorithms running on these devices analyze the data in real time and provide actionable insights to vineyard managers.
With Edge AI, vineyard managers can:
- Monitor vine health and detect diseases early on, enabling targeted interventions.
- Optimize irrigation and fertilization practices based on soil moisture and nutrient levels.
- Forecast weather conditions to prevent frost damage or plan harvest schedules.
- Improve overall crop quality and yield while reducing resource usage and operational costs.
Challenges and Future Directions
While Edge AI shows great promise for crop monitoring, there are still challenges to overcome in its widespread adoption:
- Hardware Constraints: Edge devices have limited processing power and memory, which can affect the performance of AI algorithms. Advancements in hardware technology are needed to support more complex AI models on edge devices.
- Data Quality and Diversity: Ensuring the quality and diversity of data collected by sensors is crucial for training accurate AI models. Farmers need access to reliable data sources and tools for data preprocessing and cleaning.
What's Your Reaction?
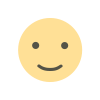
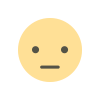
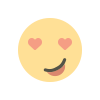
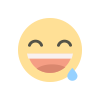
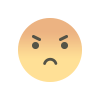
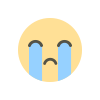
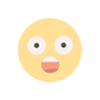