Edge AI for Commute Time Estimation
Discover how Edge AI technology is revolutionizing commute time estimation, providing real-time traffic data for more accurate travel predictions.
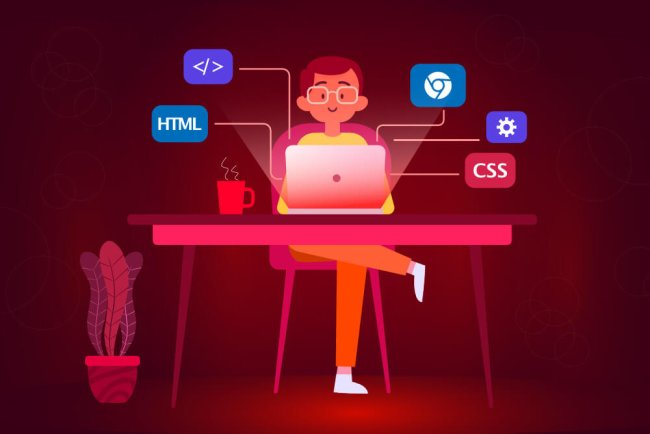
Edge AI for Commute Time Estimation
Commute time estimation is a critical aspect of transportation planning and management. In recent years, the use of Edge AI technology has revolutionized the way commute times are estimated and predicted. Edge AI refers to artificial intelligence algorithms that are deployed on local devices, such as sensors or cameras, rather than relying on a centralized cloud server. This allows for real-time processing of data and faster decision-making, making it ideal for applications like commute time estimation.
How Edge AI Works for Commute Time Estimation
Edge AI for commute time estimation involves deploying machine learning models on edge devices, such as traffic cameras, sensors, or even in-vehicle systems. These models are trained on historical traffic data to predict commute times based on various factors such as traffic flow, road conditions, weather, and time of day.
By running these models on edge devices, commute time estimation can be done in real-time without the need for constant communication with a centralized server. This allows for faster and more accurate predictions, leading to improved traffic management and better decision-making for commuters.
Benefits of Edge AI for Commute Time Estimation
There are several key benefits of using Edge AI for commute time estimation:
- Real-time Processing: Edge AI enables real-time processing of data, allowing for instant predictions of commute times. This can help commuters make informed decisions about their travel routes and timings.
- Reduced Latency: By processing data locally on edge devices, latency is reduced compared to cloud-based solutions. This ensures faster response times and more efficient traffic management.
- Improved Accuracy: Edge AI models can be continuously updated and fine-tuned based on real-time data, leading to more accurate predictions of commute times. This can help reduce congestion and optimize traffic flow.
- Privacy and Security: Edge AI processes data locally on devices, reducing the need to transmit sensitive information to a centralized server. This enhances privacy and security for commuters and ensures data protection.
Applications of Edge AI in Commute Time Estimation
Edge AI technology can be applied in various ways to improve commute time estimation:
- Traffic Monitoring: Edge AI can analyze live traffic data from cameras and sensors to predict congestion levels and estimate commute times for different routes.
- Smart Traffic Lights: Edge AI can be used to optimize traffic light timings based on real-time traffic conditions, reducing wait times and improving traffic flow.
- In-Vehicle Systems: Edge AI can be integrated into in-vehicle systems to provide personalized commute time estimates based on the driver's route preferences and real-time traffic updates.
- Public Transportation: Edge AI can analyze data from public transportation systems to predict arrival times and optimize schedules, improving the overall efficiency of the transit network.
Challenges and Considerations
While Edge AI offers many benefits for commute time estimation, there are also challenges and considerations to be aware of:
- Resource Constraints: Edge devices may have limited computational resources, which can impact the performance of AI models. Efficient model optimization and deployment are crucial to overcome resource constraints.
- Data Quality: The accuracy of commute time estimates depends on the quality of the data collected by edge devices. Ensuring data accuracy and reliability is essential for the success of Edge AI solutions.
- Privacy Regulations: Compliance with privacy regulations and data protection laws is important when deploying Edge AI solutions for commute time estimation. Ensuring data security and user privacy is a key consideration.
- Interoperability: Integration with existing transportation systems and infrastructure can be challenging. Ensuring interoperability and compatibility with different devices and platforms is necessary for seamless deployment.
What's Your Reaction?
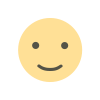
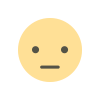
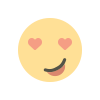
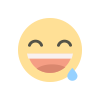
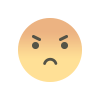
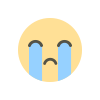
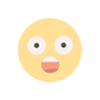