Data Analytics
Discover the power of data analytics with our comprehensive guide. Learn how to interpret, analyze, and visualize data to make informed decisions.
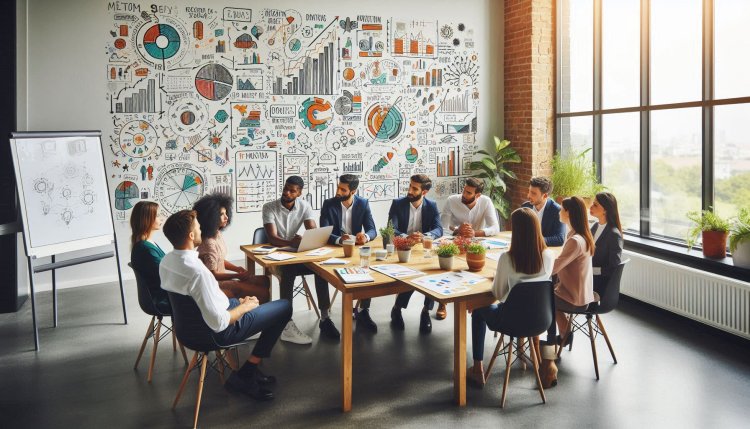
Data Analytics
Data analytics is the process of analyzing raw data to extract valuable insights and make informed decisions. It involves using various tools and techniques to interpret data, discover patterns, and uncover trends. Data analytics plays a crucial role in today's data-driven world, where organizations rely on data to drive business strategies and make data-driven decisions.
Types of Data Analytics
There are several types of data analytics that organizations can leverage to gain insights from their data:
- Descriptive Analytics: Descriptive analytics focuses on summarizing historical data to provide insights into what has happened in the past. It helps organizations understand trends, patterns, and relationships in their data.
- Diagnostic Analytics: Diagnostic analytics aims to identify the root causes of events or trends by analyzing historical data. It helps organizations understand why certain events occurred and what factors contributed to them.
- Predictive Analytics: Predictive analytics uses historical data and statistical algorithms to forecast future events or trends. It helps organizations make informed predictions and plan for the future.
- Prescriptive Analytics: Prescriptive analytics goes a step further than predictive analytics by recommending actions to optimize outcomes. It helps organizations make data-driven decisions to achieve their desired goals.
Tools and Techniques
There are various tools and techniques used in data analytics to process and analyze data:
- Statistical Analysis: Statistical analysis involves using statistical methods to analyze data and draw meaningful insights. It helps in identifying patterns, trends, and relationships in the data.
- Data Mining: Data mining is the process of discovering patterns and relationships in large datasets. It involves using algorithms to extract valuable information from raw data.
- Machine Learning: Machine learning is a subset of artificial intelligence that enables systems to learn from data and make predictions or decisions without being explicitly programmed. It is used in predictive analytics and pattern recognition.
- Text Mining: Text mining involves analyzing unstructured text data to extract valuable insights. It is used to analyze customer feedback, social media data, and other text-based sources.
- Big Data Analytics: Big data analytics involves analyzing large and complex datasets to uncover hidden patterns, correlations, and trends. It requires specialized tools and techniques to process massive amounts of data efficiently.
Benefits of Data Analytics
Data analytics offers several benefits to organizations looking to leverage their data effectively:
- Improved Decision-Making: Data analytics provides valuable insights that help organizations make informed decisions based on data-driven evidence rather than intuition.
- Enhanced Efficiency: By automating data analysis processes, data analytics helps organizations save time and resources, leading to increased efficiency.
- Better Customer Insights: Data analytics enables organizations to understand customer behavior, preferences, and trends, allowing them to tailor products and services to meet customer needs.
- Risk Mitigation: By analyzing historical data and identifying patterns, data analytics helps organizations predict and mitigate risks, such as fraud or market fluctuations.
- Competitive Advantage: Organizations that effectively leverage data analytics gain a competitive edge by making data-driven decisions and responding quickly to market changes.
Challenges of Data Analytics
While data analytics offers numerous benefits, organizations may face some challenges in implementing and leveraging data analytics effectively:
- Data Quality: Ensuring data quality is crucial for accurate analysis and decision-making. Poor data quality can lead to incorrect insights and decisions.
- Data Privacy and Security: With the increasing amount of data being collected and analyzed, organizations need to ensure the privacy and security of sensitive data to comply with regulations and protect against data breaches.
- Skills Gap: Data analytics requires specialized skills such as data science, statistics, and programming. Organizations may face challenges in finding and retaining skilled professionals to work on data analytics projects.
- Integration of Data Sources: Organizations often have data stored in different systems and formats, making it challenging to integrate and analyze data effectively. Data integration tools and techniques are essential
What's Your Reaction?
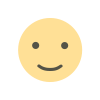
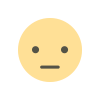
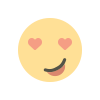
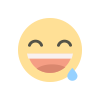
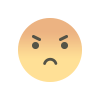
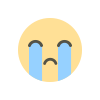
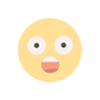