AI ethics: Bias detection and mitigation
Explore the importance of detecting and mitigating bias in AI ethics. Learn how to address ethical concerns and ensure fair and unbiased artificial intelligence systems.
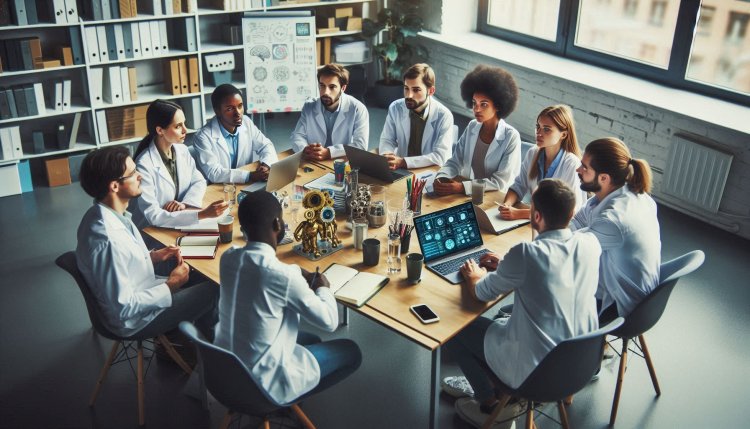
AI Ethics: Bias Detection and Mitigation
Artificial Intelligence (AI) systems have the potential to greatly benefit society by automating tasks, making processes more efficient, and providing valuable insights. However, these systems can also perpetuate and even amplify biases present in the data they are trained on. Bias in AI systems can lead to unfair outcomes, discrimination, and reinforce existing societal inequalities.
What is Bias in AI?
Bias in AI refers to the systematic and unfair preferences or prejudices that are reflected in the decisions made by AI systems. This bias can arise from the data used to train the AI models, the design of the algorithms, or the context in which the AI system is deployed.
Detecting Bias in AI
It is essential to detect bias in AI systems to ensure fair and equitable outcomes. There are several methods for detecting bias in AI:
- Dataset Analysis: Analyzing the training data to identify biases related to gender, race, age, or other sensitive attributes.
- Algorithmic Auditing: Examining the decision-making process of the AI system to understand how biases are influencing outcomes.
- Performance Disparities: Monitoring the performance of the AI system across different demographic groups to detect disparities.
- User Feedback: Collecting feedback from users to identify instances of bias in the AI system's outputs.
Mitigating Bias in AI
Once bias is detected, it is important to take steps to mitigate it. Here are some strategies for mitigating bias in AI:
- Diverse and Representative Data: Ensuring that the training data is diverse and representative of the population to reduce biases.
- Algorithmic Fairness: Incorporating fairness constraints into the AI algorithms to prevent biased outcomes.
- Regular Monitoring: Continuously monitoring the AI system's performance and re-evaluating for biases over time.
- Transparency and Explainability: Making AI systems more transparent and explainable to understand how decisions are being made.
- Human Oversight: Having human experts review and validate the AI system's outputs to ensure fairness and accountability.
Challenges in Bias Detection and Mitigation
Despite efforts to detect and mitigate bias in AI, there are several challenges that make this task complex:
- Data Quality: Biases in the training data can be subtle and hard to detect, leading to biased AI models.
- Algorithmic Complexity: AI algorithms can be complex and opaque, making it difficult to understand how biases are influencing decisions.
- Trade-offs: Mitigating bias in AI may involve trade-offs with other objectives such as accuracy or efficiency.
- Evolving Biases: Societal biases and norms change over time, requiring constant monitoring and adaptation of AI systems.
Importance of Ethical AI
Addressing bias in AI is crucial for building ethical AI systems that promote fairness, transparency, and accountability. Ethical AI ensures that AI technologies are developed and deployed in a way that respects human rights, diversity, and societal values.
Conclusion
Bias detection and mitigation are essential components of ensuring that AI systems are fair and equitable. By actively addressing biases in AI, we can build more responsible and ethical AI technologies that benefit society as a whole
What's Your Reaction?
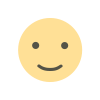
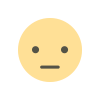
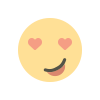
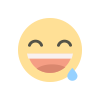
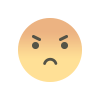
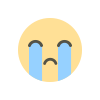
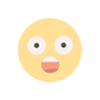