AI and renewable energy: Predictive maintenance
Explore how AI is revolutionizing renewable energy with predictive maintenance strategies, optimizing operations and reducing downtime. Learn more here.
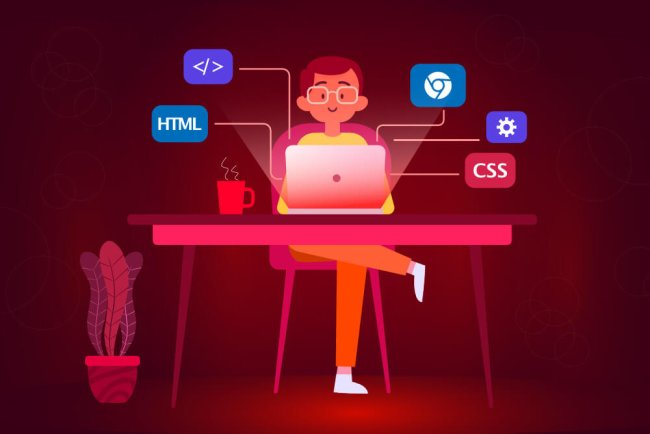
AI and Renewable Energy: Predictive Maintenance
In recent years, there has been a growing emphasis on the use of artificial intelligence (AI) in the renewable energy sector. One of the key applications of AI in this industry is predictive maintenance. Predictive maintenance involves using data and AI algorithms to predict when equipment or machinery is likely to fail, allowing for proactive maintenance to be performed and minimizing downtime. In the context of renewable energy, predictive maintenance can help ensure the reliable operation of solar panels, wind turbines, and other renewable energy assets.
Challenges in Renewable Energy
Renewable energy sources such as solar and wind power are becoming increasingly important as the world seeks to transition away from fossil fuels and reduce greenhouse gas emissions. However, these energy sources also present unique challenges when it comes to maintenance. Solar panels can be affected by factors such as weather conditions and shading, while wind turbines are subject to wear and tear from constant rotation. Traditional maintenance approaches, such as scheduled maintenance or reactive maintenance, may not be sufficient to address these challenges effectively.
Benefits of Predictive Maintenance
Predictive maintenance offers several key benefits for the renewable energy sector. By using AI algorithms to analyze data from sensors and other sources, operators can gain insights into the condition of their equipment and predict when maintenance is needed. This proactive approach can help prevent costly breakdowns and extend the lifespan of renewable energy assets. Predictive maintenance can also help optimize maintenance schedules and reduce overall maintenance costs by focusing resources on the most critical components.
How AI is Used in Predictive Maintenance
AI plays a crucial role in predictive maintenance by enabling the analysis of large amounts of data in real-time. Machine learning algorithms can be trained on historical maintenance data to identify patterns and anomalies that indicate when equipment is likely to fail. This predictive capability allows operators to take action before a failure occurs, reducing downtime and minimizing the impact on energy production.
AI can also be used to optimize maintenance schedules based on factors such as equipment condition, weather conditions, and energy demand. By taking a data-driven approach to maintenance planning, operators can ensure that maintenance activities are performed at the most opportune times, minimizing disruptions to energy production.
Case Studies
Solar Power Plants
In the solar power industry, predictive maintenance is particularly important for ensuring the efficient operation of solar panels. By monitoring factors such as panel temperature, output voltage, and shading, operators can detect issues before they lead to a drop in energy production. AI algorithms can analyze this data to predict when panels are likely to degrade or fail, allowing for timely maintenance to be performed.
Wind Farms
For wind farms, predictive maintenance can help prevent unexpected downtime and extend the lifespan of wind turbines. By analyzing data from sensors on the turbines, AI algorithms can detect early signs of wear and tear, such as vibration patterns or temperature fluctuations. This predictive capability enables operators to schedule maintenance activities proactively, ensuring that turbines remain in optimal condition for maximum energy production.
Challenges and Future Directions
While predictive maintenance offers significant advantages for the renewable energy sector, there are also challenges to be overcome. One key challenge is the availability and quality of data. In order for AI algorithms to make accurate predictions, operators need access to high-quality data from sensors and other sources. Ensuring the reliability and accuracy of this data is crucial for the success of predictive maintenance programs.
Another challenge is the integration of AI systems with existing maintenance processes and workflows. Operators may need to invest in training and infrastructure to effectively implement predictive maintenance solutions. Collaboration between data scientists, maintenance technicians, and other stakeholders is essential to ensure that AI algorithms are deployed effectively and that maintenance activities are coordinated efficiently.
Looking ahead, the future of predictive maintenance in renewable energy is bright. As AI technologies continue to advance, operators will have access to more sophisticated algorithms and tools for analyzing data and predicting equipment failures. By harnessing the power of AI, the renewable energy sector can improve the reliability and efficiency of its operations, ultimately contributing to a more sustainable energy future.
Conclusion
Predictive maintenance, powered by AI, is a valuable tool for the renewable energy sector. By using data-driven insights to predict equipment failures and optimize maintenance schedules, operators can improve the reliability and efficiency of their renewable energy assets. While there are challenges to overcome, the benefits of predictive maintenance far outweigh the costs, making it a key strategy for ensuring the long-term success of the renewable energy industry.
What's Your Reaction?
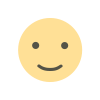
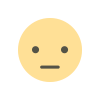
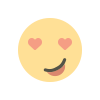
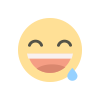
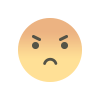
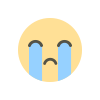
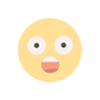